Generative AI Security Risks: 8 Critical Threats You Should Know
This blog post explores the main security risks linked to Generative AI, such as data breaches, misuse, and regulatory challenges, while also clarifying the generative AI meaning in the context of these threats. It also shows how Keepnet's Security Awareness Training can equip organizations with the tools they need to address these risks and strengthen their cybersecurity defenses.
As businesses increasingly integrate generative AI into their operations, it is significant to recognize and address the significant generative AI security risks that come with this advanced technology. Ignoring these risks can have serious consequences, such as data breaches, financial losses, and damage to a company’s reputation.
Generative AI introduces significant cybersecurity risks, leading to financial losses, operational disruptions, and reputational damage.
- In 2023, cybercriminals in Southeast Asia exploited generative AI technologies to steal up to $37 billion through various illicit activities, including romance-investment schemes and crypto fraud.
- A 2024 report revealed that 93% of organizations experienced security breaches in the past year, with nearly half reporting estimated losses exceeding $50 million, as threat actors leveraged AI for sophisticated attacks like malware creation and phishing.
- In 2024, at least five FTSE 100 companies fell victim to deepfake scams where fraudsters impersonated CEOs using AI-generated voices, leading to unauthorized fund transfers and significant reputational harm.
This alarming statistic highlights the urgent need for businesses to proactively manage and mitigate AI-related threats and understand the broader impact of generative AI.
This blog post delves into eight critical generative AI security risks that every business should be aware of and provides insights on how to effectively safeguard against these emerging threats.
What Is Generative AI?
Generative AI refers to a class of artificial intelligence models that create new content, such as text, images, music, or code, based on the data they have been trained on.
These models generate outputs that mimic the style, structure, or content of the original data, often producing novel and creative results. Understanding generative AI meaning is important, as its impact is felt across various industries. Common types of generative AI include:
- Text generation models like GPT which can write essays, answer questions, or generate code.
- Image generation models like DALL-E which can create images from textual descriptions.
- Music generation models which can compose new pieces of music.
The impact of generative AI is significant, as it is widely used in various fields, including content creation, art, design, and natural language processing.
However, as its use expands, so do the associated generative AI risks, making it significant for businesses to understand and address the potential security challenges that come with this technology.
How Does Generative AI Work?
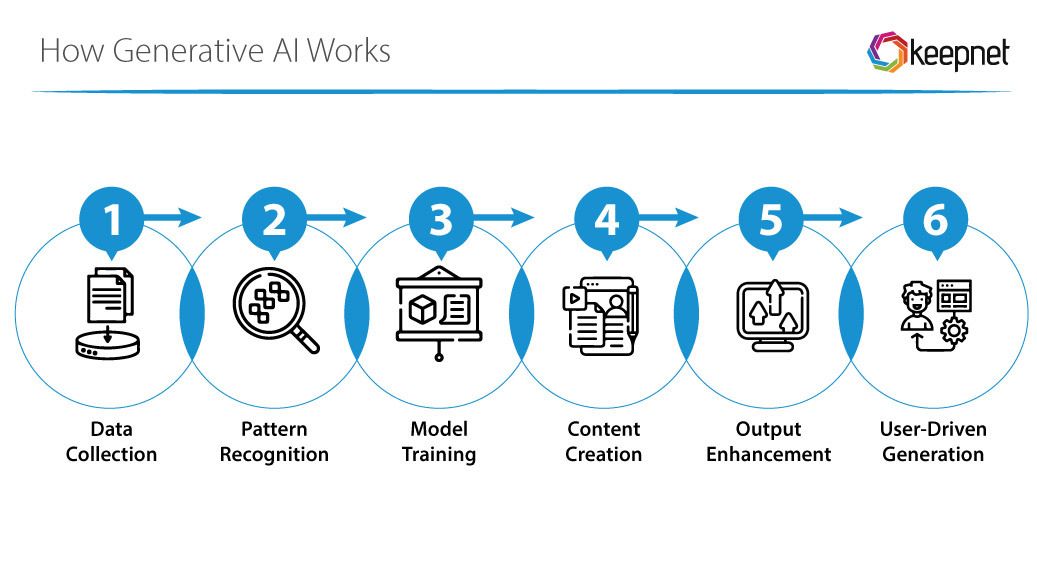
Generative AI works by using machine learning models, particularly neural networks, to learn patterns from large datasets like text, images, or audio. This process is central to what generative AI does, as it enables the AI to mimic and generate new content.
The AI is first trained on this data, analyzing and identifying patterns, such as sentence structure in text or color composition in images.
Once trained, the model can generate new content that closely resembles the original data by predicting what should come next in a sequence or creating entirely new examples based on what it has learned.
This ability to produce realistic and high-quality results is a key aspect of the impact of generative AI. The model continuously improves as it processes more data, refining its output through simpler techniques that allow it to consistently generate high-quality content.
Understanding how generative AI works is significant for leveraging its potential while also being aware of the generative AI risks that come with its use.
What Is The Impact of Generative AI on Overall Cybersecurity?
The impact of generative AI on cybersecurity is significant and has both positive and negative effects. On the positive side, generative AI can enhance cybersecurity by identifying vulnerabilities, generating defensive code, and simulating potential attacks to improve defenses.
It also helps create stronger security protocols and automates threat detection and response, making systems more resilient against generative AI security risks.
On the negative side, generative AI can be misused by cybercriminals to develop more sophisticated phishing attacks, create advanced malware, or bypass security measures. It can also enable the automation of large-scale attacks, increasing the frequency and complexity of cyber threats.
The rise of generative AI and the associated generative AI risks demand stronger cybersecurity measures to address these emerging challenges and protect against potential threats.
8 Security Risks of Generative AI
Generative AI brings several security risks that organizations must carefully consider. First, there's the risk of sensitive data being exposed through AI-generated outputs, a significant generative AI risk.
Additionally, generative AI can be misused for harmful purposes, such as creating misleading content or spreading disinformation, which further illustrates the impact of generative AI on cybersecurity. Another concern is the potential for AI models to be stolen or copied, compromising valuable intellectual property.
Bias and discrimination can also be issues, with generative AI systems unintentionally reinforcing harmful stereotypes or making unfair decisions.
These systems are also vulnerable to being tricked into making incorrect decisions, raising concerns about their reliability in unexpected situations.
Furthermore, the lack of transparency in how AI makes decisions complicates trust and accountability. Lastly, keeping up with evolving legal and ethical standards adds an extra layer of complexity. We will explore each of these risks in more detail to better understand their effects.
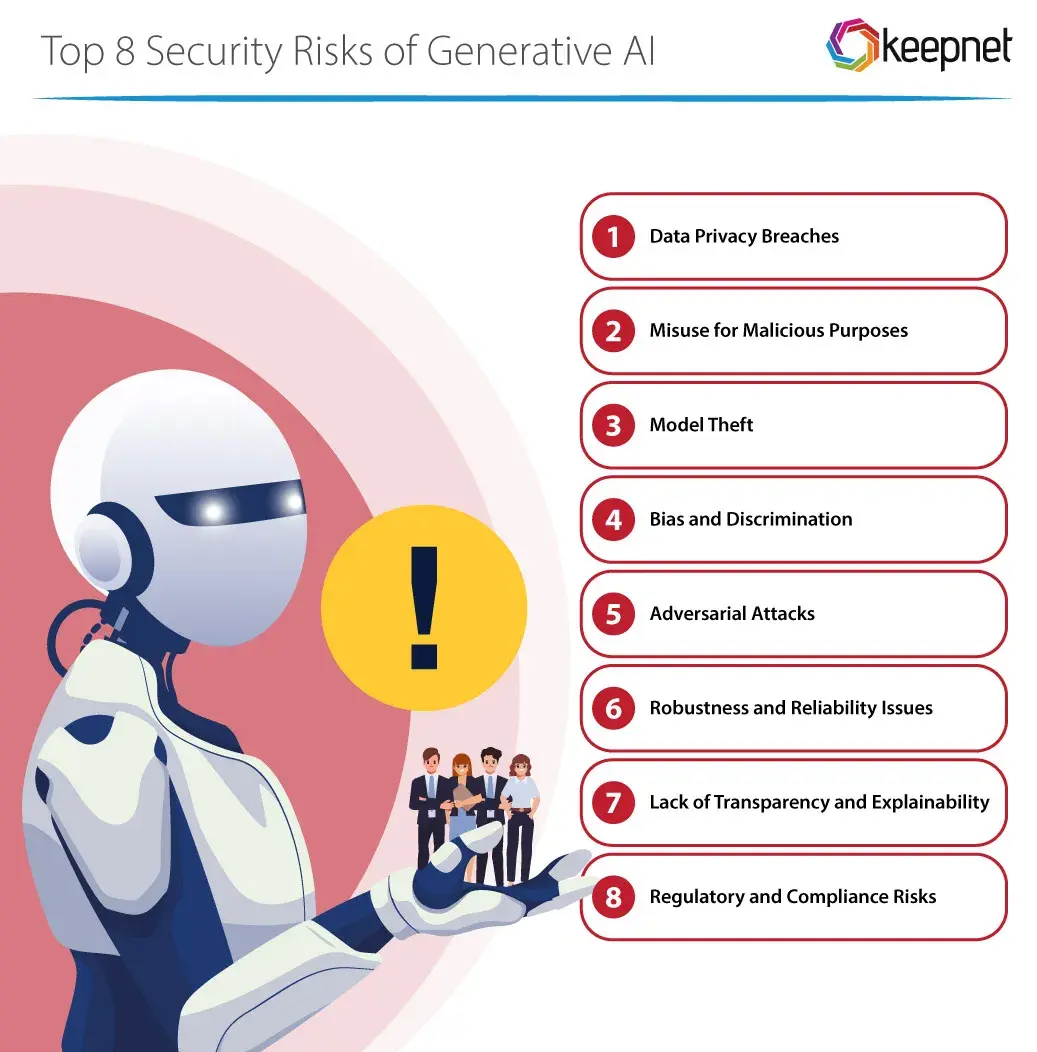
Data Privacy Breaches
Generative AI carries significant data privacy risks because it processes and generates content from large datasets that might include sensitive information.
During training, AI models can unintentionally produce outputs that expose private or confidential data, such as reproducing parts of confidential documents or recreating identifiable faces from the training data.
This risk is especially concerning in applications like chatbots or virtual assistants, where real-time user data is involved.
To reduce these risks, organizations should anonymize training data, use secure data handling practices, and regularly check AI outputs for accidental data leaks.
Strong data governance and transparency with users are important to preventing data privacy breaches in generative AI.
Misuse for Malicious Purposes
Generative AI can be misused for malicious purposes, posing serious security risks. One of the most concerning threats is the creation of deepfakes—highly realistic but fake images, videos, or audio recordings that can be used to spread disinformation, manipulate public opinion, or blackmail individuals.
Cybercriminals can also use generative AI to craft more convincing phishing emails or social engineering attacks, making it easier to deceive and exploit victims. Additionally, AI-generated code can be manipulated to develop sophisticated malware or automate large-scale cyberattacks.
The accessibility of generative AI tools further increases these risks, as they enable even non-experts to create harmful content.
To mitigate these threats, it is significant to implement strict regulations, monitor the use of AI technologies, and develop countermeasures that can detect and prevent the misuse of generative AI for malicious purposes.
Watch the video below, where Keepnet demonstrates a real-life example of a sophisticated AI-driven vishing scam, showcasing the advanced tactics used in this type of social engineering attack.
Model Theft
Model theft is a significant risk in the field of generative AI, where unauthorized individuals or entities can steal, replicate, or misuse AI models.
These models often represent a substantial investment of time, resources, and expertise, making them valuable intellectual property for organizations.
If a model is stolen, it can be used by competitors to gain an unfair advantage or by malicious actors to create harmful applications, such as generating disinformation or creating advanced cyber threats.
Moreover, once a model is stolen, it can be reverse-engineered, allowing the thief to extract sensitive data or proprietary techniques embedded within the model.
This not only undermines the security and privacy of the data used to train the model but also compromises the competitive edge of the organization that developed it.
To protect against model theft, it is crucial to implement robust security measures, such as encryption, access controls, and monitoring, as well as legal safeguards like patents and copyright protections. These steps help ensure that AI models remain secure and that their use is controlled and ethical.
Bias and Discrimination
Bias and discrimination are major concerns when it comes to generative AI risks. These systems can unintentionally pick up and reinforce existing biases in the data they are trained on.
If an AI model learns from biased data, it might produce unfair or biased outcomes, such as favoring certain groups over others in decision-making processes like hiring. For example, if a generative AI used for job screening is trained on data that reflects past biases, it might unfairly disadvantage certain demographics.
Similarly, AI-generated content could include stereotypes or discriminatory language without intending to. These issues can lead to real-world harm by reinforcing social inequalities or negative stereotypes.
To prevent this, it's important to carefully select and process training data to reduce bias, regularly check AI models for fairness, and monitor the content they produce. Including diverse perspectives in the development of AI systems is also key to identifying and addressing potential biases.
By taking these precautions, organizations can help ensure that generative AI promotes fair and equitable outcomes.
Adversarial Attacks
Adversarial attacks are a significant threat in the context of generative AI, where malicious actors intentionally manipulate input data to trick or mislead AI models.
These attacks involve creating slightly altered inputs, such as modified images or text, that appear normal to humans but cause the AI to make incorrect or harmful decisions.
For example, an adversarial attack might trick an AI model into misclassifying an image, interpreting a stop sign as a speed limit sign, which could have serious consequences in autonomous driving systems.
In the case of generative AI, adversarial attacks can lead to the creation of misleading or harmful content, undermining the reliability and safety of the AI’s outputs. These attacks exploit vulnerabilities in the AI model’s learning process, revealing weaknesses that can be targeted by cybercriminals.
To defend against adversarial attacks, it is crucial to develop robust AI models that can withstand such manipulations, regularly test models with adversarial scenarios, and implement security measures that detect and prevent these types of attacks. By doing so, organizations can protect the integrity and trustworthiness of their generative AI systems.
Robustness and Reliability Issues
Ensuring robustness and reliability in generative AI is a significant challenge, as these models can sometimes struggle when faced with unexpected or unfamiliar inputs. Although generative AI models are powerful, they can produce unpredictable or incorrect results, especially when dealing with scenarios outside their training data.
This lack of robustness can result in unreliable outputs, potentially leading to harmful or misleading outcomes in practical applications.
For example, an AI model used to generate legal documents might produce inaccurate or nonsensical text when encountering unfamiliar situations, leading to serious legal or financial risks.
Moreover, the model’s sensitivity to minor changes in input data can result in inconsistent and untrustworthy outputs.
To overcome these issues, it is essential to conduct thorough testing, maintain ongoing monitoring, and implement fail-safes to ensure that the AI performs reliably across diverse scenarios. Without addressing these robustness and reliability concerns, the effectiveness and safety of generative AI in critical tasks could be significantly compromised.
Lack of Transparency and Explainability
A key issue with generative AI is its lack of transparency and explainability, making it challenging to understand how these models produce their outputs. Generative AI, particularly those utilizing deep learning techniques, often operates in a "black box" manner, where the decision-making process is hidden and difficult to interpret.
This lack of clarity can lead to trust issues, especially in high-stakes fields like healthcare, finance, and legal services, where understanding the reasoning behind decisions is crucial. Without transparency, it’s hard to assess the reliability of the AI's outcomes or identify potential biases and errors.
This can result in unintended consequences or misuse of the technology. To build trust and ensure ethical use, it is important to develop ways to make AI processes more understandable, allowing users to see and trust the reasons behind the AI's decisions.
Regulatory and Compliance Risks
Navigating regulatory and compliance challenges is a critical concern when deploying generative AI systems. As these technologies rapidly evolve, laws and regulations struggle to keep pace, creating a complex legal landscape.
Organizations using generative AI must navigate various regulations related to data privacy, intellectual property, and ethical use, which can vary widely across different regions and industries. Failure to comply with these regulations can result in legal penalties, financial losses, and damage to the organization’s reputation.
Moreover, the lack of clear guidelines on AI use can lead to uncertainty, making it difficult for companies to ensure their AI practices are fully compliant.
This uncertainty is made worse by the possibility that AI-generated content could break copyright laws, ignore data protection rules, or spread biased or harmful information, which increases regulatory risks even more.
To reduce these risks, organizations need to stay informed about evolving regulations, implement strong compliance strategies, and ensure that their AI systems are designed and operated within legal and ethical boundaries.
Regular communication with regulators and following best practices can help organizations avoid legal issues and maintain trust with their stakeholders.
Protect of Generative AI Security Risks with Keepnet's Security Awareness Training
Keepnet helps organizations effectively manage the security risks associated with generative AI through its tailored security awareness training program.
As AI-driven threats become more sophisticated, Keepnet’s platform is designed to build a strong security culture that can counter these emerging challenges.
Key features of Keepnet Security Awareness Training that specifically address generative AI security risks include:
- Behavior-Based Training: Phishing simulators across various platforms (Vishing, smishing, QR phishing) correct user mistakes in real time, helping to prevent breaches that AI-generated attacks could exploit.
- Security Training Marketplace: Access to over 2000 training modules from 12 providers ensures that the content is always up-to-date and relevant to the latest AI-related threats.
- Automated and Customizable Content: Keepnet adapts training based on observed behaviors, offering a proactive and personalized approach to mitigating AI-driven risks.
Keepnet empowers your organization to mitigate the unique security risks posed by generative AI, creating a customized training program that equips your employees to effectively recognize and respond to these new challenges.
Watch the video below to see how Keepnet Security Awareness Training can strengthen your organization’s defenses and empower your team to confidently handle the security challenges posed by generative AI.
Editor's Note: This article was updated on February 14, 2025.